Churn is one of the most crucial metrics for any game or app developer. Churn is a silent killer for games: while user acquisition efforts often focus on growing the user base, churn can quickly counteract any gains if not managed properly.
Understanding churn goes beyond just knowing how many users leave. It’s about identifying when and why users leave, which can help shape product development and marketing strategies. By analyzing churn, developers can take proactive steps to reduce its impact, making it a crucial metric for the health of any game.
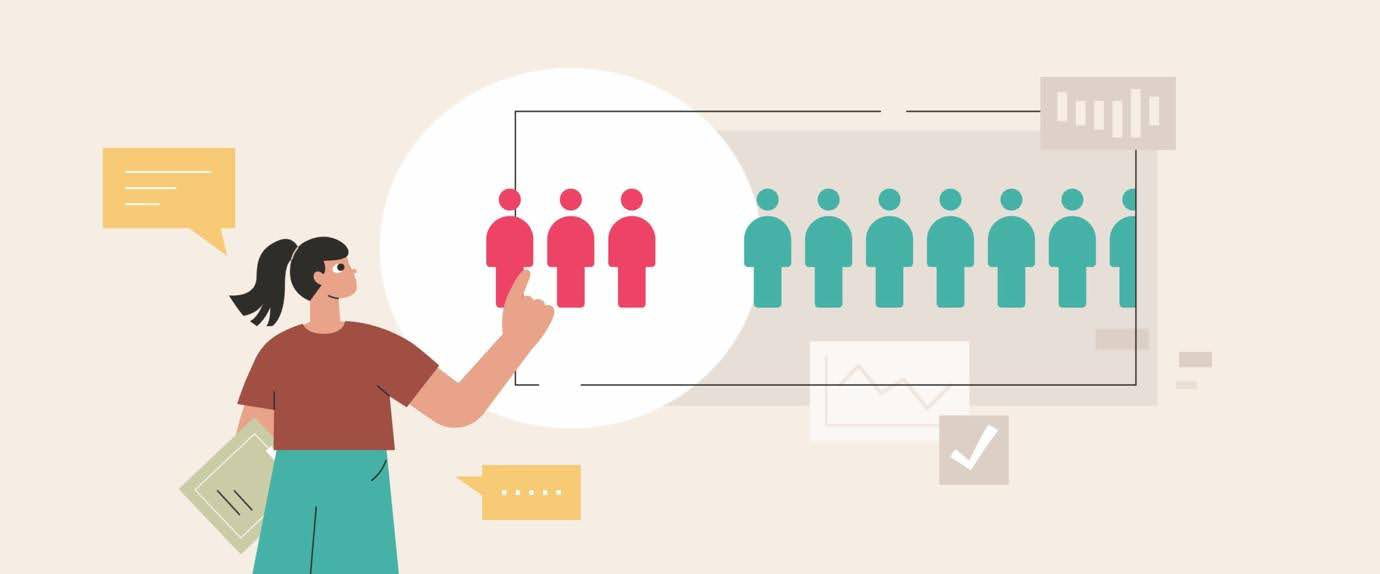
The Importance of Predicting Churn
Predicting churn and understanding reasons behind it are essential for implementing effective user retention strategies. While preventing all users from leaving is impossible, identifying those at risk allows developers to intervene proactively. Early detection enables targeted strategies to re-engage users, optimizing retention and enhancing lifetime value.
Churn can be extremely costly for mobile games and apps with high user acquisition costs and a high CPI. When users churn early, businesses lose not only the money spent on acquiring them but also the potential revenue they could have generated through IAPs or ad views. The reality is that reducing churn is typically more cost-effective than constantly acquiring new users. It’s often cheaper to keep a user than to acquire a new one, which makes churn prediction an invaluable tool in ensuring better long-term profitability and a higher return on investment.
All companies dream of taking the right steps to prevent churn and keep users in their app/game. However, predicting churn is not without its challenges. From a technical standpoint, this is a challenging issue, which is why prediction is necessary. This represents a technological step that often intimidates game and app companies. In fact, there are several factors that make churn prediction a difficult yet essential task. To explore these challenges, as well as how advanced techniques such as machine learning can help, let’s dive deeper into the complexities of churn prediction.
In the remainder of this blog post, we will explore two distinct types of churn that need to be addressed: early and late funnel churn. We will also discuss the strategies and approaches that can be applied to predict and tackle these two churn funnel segments effectively.
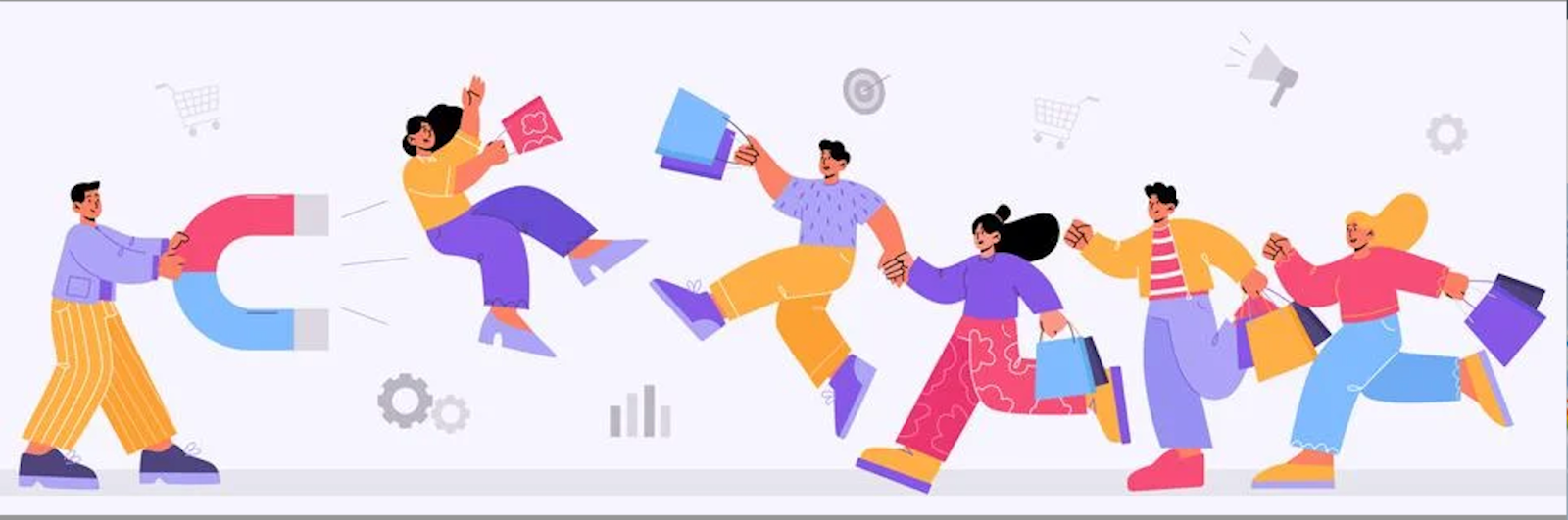
Early Funnel Churn
Early funnel churn is a significant challenge for mobile game and app developers, especially when investing heavily in marketing to attract new users. If players leave too soon, they don’t generate enough revenue through ads or in-app purchases to offset marketing costs, directly impacting profitability and putting pressure on teams. Identifying churn risks early allows you to optimize onboarding, enhance rewards, and create a more engaging experience to retain users and improve your ROAS.
Predicting early funnel churn and identifying its causes allow you to implement real-time strategies that boost retention and monetization. If users churn too early, reaching ROAS goals becomes significantly harder. Understanding these bottlenecks is crucial for optimizing your engagement and IAP strategies.
Analyzing the reasons behind early churn and implementing targeted strategies will help you achieve sustainable growth, ensuring that your marketing efforts deliver stronger results in the long run.
Let’s also discuss how late funnel churn impacts the long term profitability and longevity of games & apps.
Late Funnel Churn
Late funnel churn refers to the point in the user journey when players or users, who have already passed through the initial stages and invested time in the game or app, decide to stop engaging. Unlike early funnel churn, where users drop off quickly due to poor onboarding or lack of immediate engagement, late funnel churn often involves users who have formed a deeper connection with the product but eventually lose interest or find reasons to disengage.
This unpredictability also leads to another problem: developers often don’t know what actions to take to prevent churn among these users. Since late-stage users are already familiar with the game, traditional methods of re-engagement, like offering incentives or pushing updates, may no longer be effective. Without clear insight into why these users are losing interest, developers struggle to craft targeted strategies that can reignite their engagement.
For established games, one major problem appears as churn of long term whale players. The loss of high-value users can significantly impact profitability, as they contribute a large portion of revenue. Predicting whale churn and offering personalized incentives are crucial strategies to retain these valuable players and safeguard long-term revenue.
How Machine Learning Helps Predict Churn
Machine learning has become a critical tool for predicting churn. By leveraging historical user data, ML algorithms can learn to recognize patterns in user behavior that precede churn. These algorithms can then predict which users are most likely to leave based on factors such as play frequency, in-app activity, session length, and interactions with social features.
By identifying users at risk, developers can then take timely action, such as sending targeted offers, new content, or notifications, to re-engage them. This proactive approach, driven by ML predictions, helps reduce churn and ensures users feel valued and more connected to the experience.
Moreover, by using sophisticated algorithms, developers can not only predict who might churn but also why they might do so, allowing for better strategies for retention.
How AppNava Offers Solutions to Churn Prediction
AppNava is a prediction suite providing ML powered user level predictions and analysis. Among many prediction offerings, churn prediction and analysis are popular prediction services utilized by many apps & games. By using advanced ML techniques, our platform enables developers to analyze user behavior, identify at-risk users, and take steps to reduce churn before it happens.
Applying ML powered churn predictions help game and app companies surpass the limitation of traditional methods. Traditionally game companies define churn with rule based systems, resulting in late identification and action. With the help of advanced ML models, AppNava can provide churn predictions as early as the first session.
For both early and late funnel churn, there are different prediction models and strategies implemented to provide the best results. In addition, AppNava offers real-time insights into user engagement, highlighting behavior trends and providing data that helps to reduce churn rates. It offers detailed analytics on user segmentation and churn reasons. By focusing on these key aspects, AppNava helps developers not only predict churn but also create strategies that keep users engaged for longer periods.
One of the key differentiators of AppNava is its ability to continuously optimize its churn prediction models as more user-level data is collected. This ensures that the platform evolves alongside user behavior patterns, making the predictions more accurate over time. This level of adaptability enables developers to stay ahead of churn trends, ensuring a more sustainable user base.
If you are struggling with high churn rates, ambiguous churn reasons and wasted marketing budgets due to retention problems, we are here to help you! Book a call to learn more about how we can customize our prediction suite for your needs.
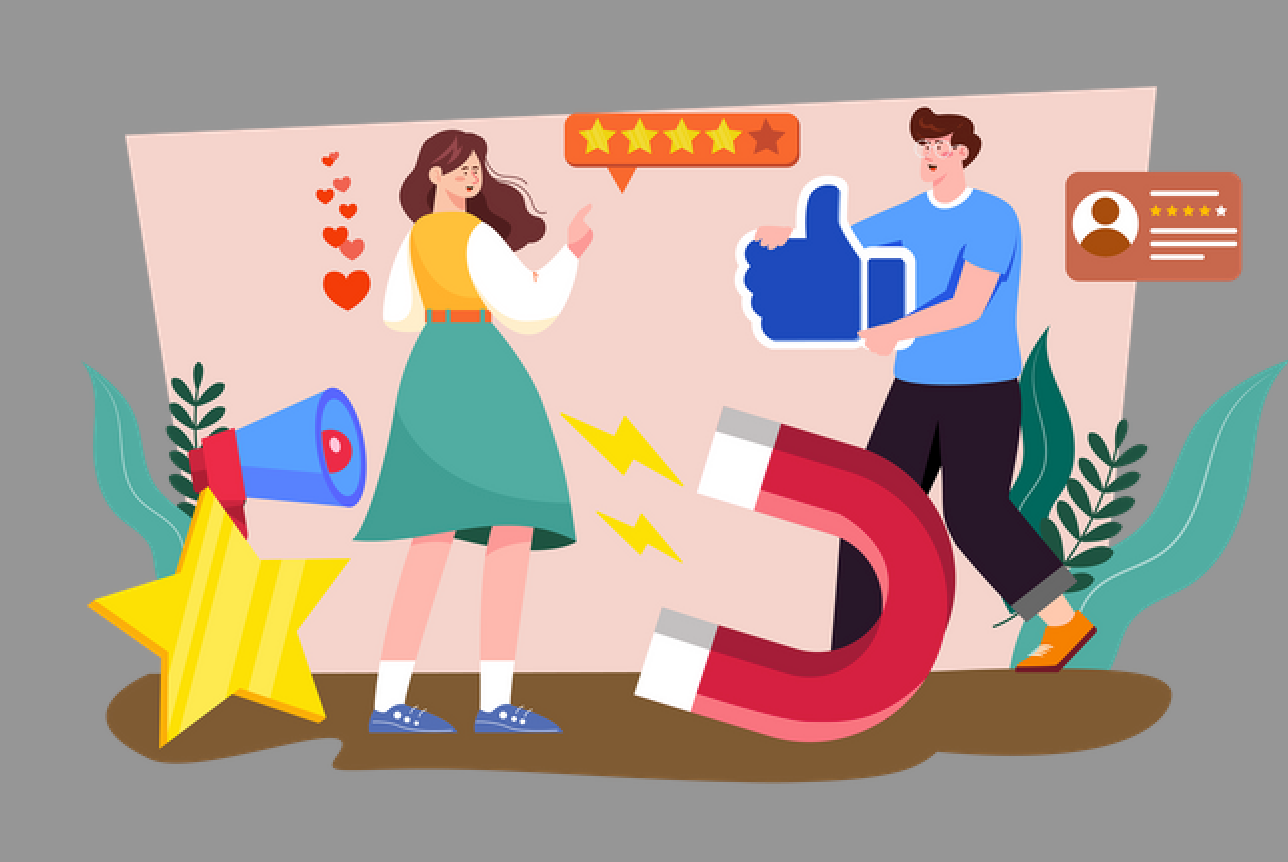